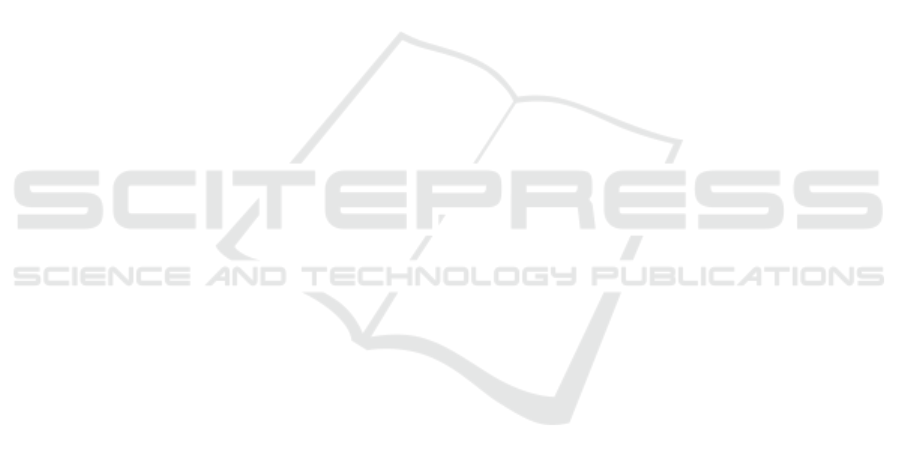
Ji, L., Wang, Y., Shi, B., Zhang, D., Wang, Z., and Yan,
J. (2019). Microsoft concept graph: Mining semantic
concepts for short text understanding. Data Intelli-
gence, 1(3):238–270.
Kadhim, A. I. (2019). Survey on supervised machine learn-
ing techniques for automatic text classification. Artifi-
cial Intelligence Review, 52(1):273–292.
Kieckbusch, D. S., Geraldo Filho, P., Di Oliveira, V., and
Weigang, L. (2021). Scan-nf: A cnn-based system for
the classification of electronic invoices through short-
text product description.
Kowsari, K., Jafari Meimandi, K., Heidarysafa, M., Mendu,
S., Barnes, L., and Brown, D. (2019). Text classifica-
tion algorithms: A survey. Information, 10(4):150.
Lake, B. M. and Murphy, G. L. (2021). Word meaning in
minds and machines. Psychological review.
Lucena, L. F., de Menezes e Silva Filho, T., do R
ˆ
ego, T. G.,
and Malheiros, Y. (2022). Automatic recognition of
units of measurement in product descriptions from
tax invoices using neural networks. In Pinheiro, V.,
Gamallo, P., Amaro, R., Scarton, C., Batista, F., Silva,
D., Magro, C., and Pinto, H., editors, Computational
Processing of the Portuguese Language, pages 156–
165, Cham. Springer International Publishing.
Luo, Z., Huang, S., and Zhu, K. Q. (2019). Knowledge
empowered prominent aspect extraction from prod-
uct reviews. Information Processing & Management,
56(3):408–423.
Marinho, M., Oliveira, V., Neto, S., Weigang, L., and
Borges, V. (2022). Visual Analysis of Electronic In-
voices to Identify Suspicious Cases of Tax Frauds,
chapter ICITS 2022 Lecture Notes in Networks and
Systems, pages 185–195. Springer.
Maulud, D. H., Zeebaree, S. R., Jacksi, K., Sadeeq, M.
A. M., and Sharif, K. H. (2021). State of art for seman-
tic analysis of natural language processing. Qubahan
Academic Journal, 1(2):21–28.
McAuley, J. and Leskovec, J. (2013). From amateurs to
connoisseurs: Modeling the evolution of user exper-
tise through online reviews. International World Wide
Web Conference Committee IW3C2.
Mendes Thame Denny, D., Ferreira Paulo, R., and Crespo
Queiroz Neves, F. (2021). Technological alternative
to tax compensation (icms credits): Case study of the
feasibility of using dlt in the brazilian electronic in-
voice system. Braz. J. Pub. Pol’y, 11:520.
Minaee, S., Kalchbrenner, N., Cambria, E., Nikzad, N.,
Chenaghlu, M., and Gao, J. (2021). Deep learning–
based text classification: a comprehensive review.
ACM Computing Surveys (CSUR), 54(3):1–40.
Oh, S. J., Schiele, B., and Fritz, M. (2019). Towards
reverse-engineering black-box neural networks. In
Explainable AI: Interpreting, Explaining and Visual-
izing Deep Learning, pages 121–144. Springer.
Pandolfo, L. and Pulina, L. (2021). Arkivo dataset: A
benchmark for ontology-based extraction tools.
Pintas, J. T., Fernandes, L. A., and Garcia, A. C. B. (2021).
Feature selection methods for text classification: a
systematic literature review. Artificial Intelligence Re-
view, 54(8):6149–6200.
Pipicano, E. F. M., Arias-Rojas, W., dos Santos, E. M., and
Fonseca, A. P. (2021). Roterisation allocation for ur-
ban freight transport, using data from electronic tax
invoices: Case study federal district of brazil. Journal
of Tianjin University Science and Technology, 54(11).
Raghavan, P. and El Gayar, N. (2019). Fraud detection us-
ing machine learning and deep learning. In 2019 in-
ternational conference on computational intelligence
and knowledge economy (ICCIKE), pages 334–339.
IEEE.
Schulte, J., Giuntini, F., Nobre, R., Nascimento, K.,
Meneguette, R., Weigang, L., Gonc¸alves, V., and
Filho, G. (2022). Elinac: Autoencoder approach for
electronic invoices data clustering. Applied Sciences,
12:3008.
Shi, Q., Wang, Y., Sun, J., and Fu, A. (2018). Short text un-
derstanding based on conceptual and semantic enrich-
ment. In International Conference on Advanced Data
Mining and Applications, pages 329–338. Springer.
Sinayobye, J. O., Kiwanuka, F., and Kyanda, S. K.
(2018). A state-of-the-art review of machine learn-
ing techniques for fraud detection research. In 2018
IEEE/ACM symposium on software engineering in
africa (SEiA), pages 11–19. IEEE.
Sokol, K. and Flach, P. (2020). Explainability fact sheets:
a framework for systematic assessment of explainable
approaches. In Proceedings of the 2020 Conference
on Fairness, Accountability, and Transparency, pages
56–67.
Suchanek, F. M., Kasneci, G., and Weikum, G. (2008).
Yago: A large ontology from wikipedia and wordnet.
Journal of Web Semantics, 6(3):203–217.
Sugrim, S. (2020). Robust models and evaluation for sys-
tems security research. PhD thesis, Rutgers The State
University of New Jersey, School of Graduate Studies.
Tang, Z., Dai, D., Chen, Z., and Chen, T. (2022). Short
text classification combining keywords and knowl-
edge. In 2022 2nd International Conference on Con-
sumer Electronics and Computer Engineering (IC-
CECE), pages 662–665.
Thangaraj, M. and Sivakami, M. (2018). Text classifi-
cation techniques: a literature review. Interdisci-
plinary Journal of Information, Knowledge, and Man-
agement, 13:117.
Vieira, P. A., Pimenta, D. P., Cruz, A. F. d., and Souza, E.
M. S. d. (2019). Effects of the electronic invoice pro-
gram on the increase of state collection. Revista de
Administrac¸
˜
ao P
´
ublica, 53:481–491.
Wu, W., Li, H., Wang, H., and Zhu, K. Q. (2012). Probase:
A probabilistic taxonomy for text understanding. In
Proceedings of the 2012 ACM SIGMOD International
Conference on Management of Data, pages 481–492.
Zimbra, D., Abbasi, A., Zeng, D., and Chen, H. (2018). The
state-of-the-art in twitter sentiment analysis: A review
and benchmark evaluation. ACM Trans. Manage. Inf.
Syst., 9(2).
WEBIST 2022 - 18th International Conference on Web Information Systems and Technologies
264