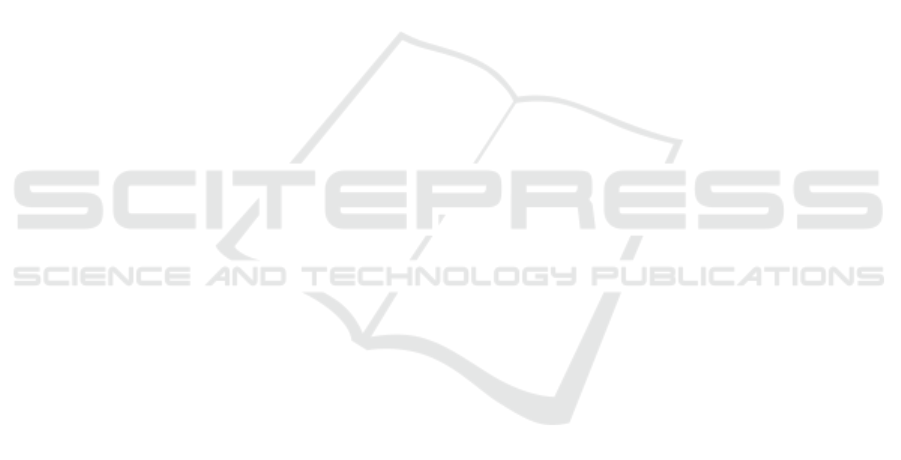
types. In Journal of Physics: Conference Series, vol-
ume 1963, page 012173. IOP Publishing.
Nielsen, M. A. (2015). Neural networks and deep learning,
volume 25. Determination press San Francisco, USA.
Pan, S. J., Tsang, I. W.-H., Kwok, J. T.-Y., and Yang, Q.
(2011). Domain adaptation via transfer component
analysis. IEEE Transactions on Neural Networks,
22:199–210.
Pan, S. J. and Yang, Q. (2010). A survey on transfer learn-
ing. IEEE Transactions on Knowledge and Data En-
gineering, 22(10):1345–1359.
Pappagari, R., Villalba, J., and Dehak, N. (2018). Joint
verification-identification in end-to-end multi-scale
cnn framework for topic identification. In 2018 IEEE
International Conference on Acoustics, Speech and
Signal Processing (ICASSP), pages 6199–6203.
Park, Y. and Gates, S. C. (2009). Towards real-time
measurement of customer satisfaction using automat-
ically generated call transcripts. In Proceedings of
the 18th ACM Conference on Information and Knowl-
edge Management, CIKM ’09, page 1387–1396, New
York, USA. Association for Computing Machinery.
Parloff, R. (2016). Why deep learning is suddenly changing
your life. Fortune. New York: Time Inc.
Pascanu, R., Mikolov, T., and Bengio, Y. (2013). On the dif-
ficulty of training recurrent neural networks. In Pro-
ceedings of the 30th International Conference on Int.
Conf. on Machine Learning - Volume 28, ICML’13,
page III–1310–III–1318. JMLR.org.
Pennington, J., Socher, R., and Manning, C. (2014). GloVe:
Global vectors for word representation. In Proceed-
ings of the 2014 Conference on Empirical Methods in
Natural Language Processing (EMNLP), pages 1532–
1543, Doha, Qatar. Association for Computational
Linguistics.
Peters, M. E., Neumann, M., Iyyer, M., Gardner, M., Clark,
C., Lee, K., and Zettlemoyer, L. (2018). Deep contex-
tualized word representations.
Radford, A., Narasimhan, K., Salimans, T., and Sutskever,
I. (2018). Improving language understanding by gen-
erative pre-training.
Raina, R., Battle, A., Lee, H., Packer, B., and Ng, A. Y.
(2007). Self-taught learning: Transfer learning from
unlabeled data. In Proceedings of the 24th Int. Conf.
on Machine Learning, ICML ’07, page 759–766, New
York, USA. Association for Computing Machinery.
Ruder, S., Peters, M. E., Swayamdipta, S., and Wolf, T.
(2019). Transfer learning in natural language process-
ing. In Proceedings of the 2019 Conf. of the North
American Chapter of the Association for Computa-
tional Linguistics: Tutorials, pages 15–18, Minneapo-
lis, USA. Association for Computational Linguistics.
Seo, M., Min, S., Farhadi, A., and Hajishirzi, H. (2017).
Neural speed reading via skim-rnn.
Shen, D., Zhang, Y., Henao, R., Su, Q., and Carin, L.
(2018). Deconvolutional latent-variable model for text
sequence matching. Proceedings of the AAAI Confer-
ence on Artificial Intelligence, 32(1).
Silva, B., Alves, J., Rebeschini, J., Querol, D., Pereira, E.,
and Celestino, V. (2021). Data science applied to fi-
nancial products portfolio. In Anals of Meeting of Na-
tional Association of Post-graduation and Research in
Administration.
Sree, K. and Bindu, C. (2018). Data analytics: Why data
normalization. International Journal of Engineering
and Technology (UAE), 7:209–213.
Sun, C., Qiu, X., Xu, Y., and Huang, X. (2019). How
to fine-tune bert for text classification? In Sun,
M., Huang, X., Ji, H., Liu, Z., and Liu, Y., editors,
Chinese Computational Linguistics, pages 194–206,
Cham. Springer International Publishing.
Thompson, N. C., Greenewald, K., Lee, K., and Manso,
G. F. (2020). The computational limits of deep learn-
ing.
van den Bulk, L. M., Bouzembrak, Y., Gavai, A., Liu, N.,
van den Heuvel, L. J., and Marvin, H. J. (2022). Auto-
matic classification of literature in systematic reviews
on food safety using machine learning. Current Re-
search in Food Science, 5:84–95.
van Dinter, R., Catal, C., and Tekinerdogan, B. (2021). A
decision support system for automating document re-
trieval and citation screening. Expert Systems with Ap-
plications, 182:115261.
Vaswani, A., Shazeer, N., Parmar, N., Uszkoreit, J.,
Jones, L., Gomez, A. N., Kaiser, L., and Polo-
sukhin, I. (2017). Attention is all you need. CoRR,
abs/1706.03762.
Wang, G., Li, C., Wang, W., Zhang, Y., Shen, D., Zhang,
X., Henao, R., and Carin, L. (2018). Joint embedding
of words and labels for text classification.
Weigang, L. (1998). A study of parallel self-organizing
map. arXiv preprint quant-ph/9808025.
Weigang, L. and da Silva, N. C. (1999). A study of paral-
lel neural networks. In IJCNN’99. International Joint
Conference on Neural Networks. Proceedings (Cat.
No. 99CH36339), volume 2, pages 1113–1116. IEEE.
Weigang, L., Enamoto, L. M., Li, D. L., and Rocha Filho,
G. P. (2022). New directions for artificial intelli-
gence: Human, machine, biological, and quantum in-
telligence. Frontiers of Information Technology &
Electronic Engineering, 23(6):984–990.
Xiao, L., Wang, G., and Zuo, Y. (2018). Research on
patent text classification based on word2vec and lstm.
In 2018 11th International Symposium on Computa-
tional Intelligence and Design (ISCID), volume 01,
pages 71–74.
Yang, Z., Yang, D., Dyer, C., He, X., Smola, A., and Hovy,
E. (2016). Hierarchical attention networks for docu-
ment classification. In Proceedings of the 2016 Con-
ference of the North American Chapter of the Associa-
tion for Computational Linguistics: Human Language
Technologies, pages 1480–1489, San Diego, Califor-
nia. Association for Computational Linguistics.
Yogatama, D., Dyer, C., Ling, W., and Blunsom, P. (2017).
Generative and discriminative text classification with
recurrent neural networks.
Zhang, D., Xu, H., Su, Z., and Xu, Y. (2015). Chinese
comments sentiment classification based on word2vec
and svmperf. Expert Systems with Applications,
42(4):1857–1863.
WEBIST 2022 - 18th International Conference on Web Information Systems and Technologies
212