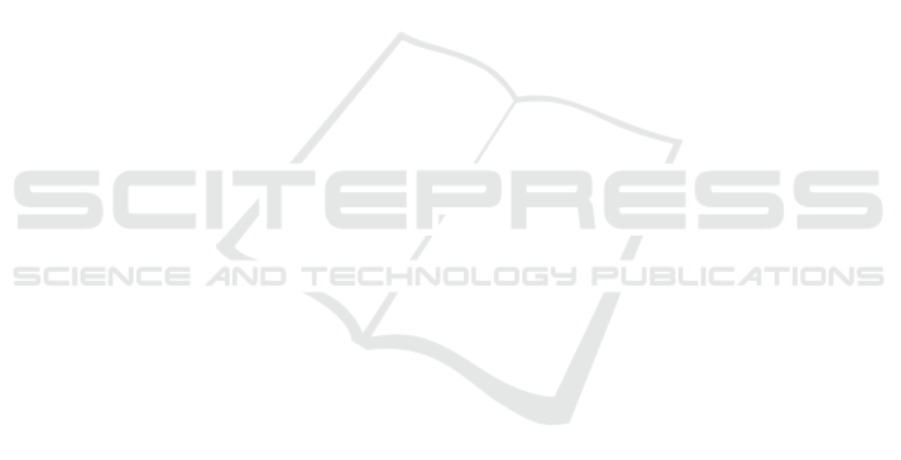
evaluating motivational interviewing fidelity via sta-
tistical text classification. Implementation Science,
9(1):1–11.
Bird, S., Dud
´
ık, M., Edgar, R., Horn, B., Lutz, R., Milan,
V., Sameki, M., Wallach, H., and Walker, K. (2020).
Fairlearn: A toolkit for assessing and improving fair-
ness in ai. Microsoft, Tech. Rep. MSR-TR-2020-32.
Cao, J., Tanana, M., Imel, Z., Poitras, E., Atkins, D., and
Srikumar, V. (2019). Observing dialogue in therapy:
Categorizing and forecasting behavioral codes. In
Proceedings of the 57th Annual Meeting of the As-
sociation for Computational Linguistics, pages 5599–
5611.
Chouldechova, A. and Roth, A. (2020). A snapshot of the
frontiers of fairness in machine learning. Communi-
cations of the ACM, 63(5):82–89.
Dess
`
ı, D., Helaoui, R., Kumar, V., Recupero, D. R., and
Riboni, D. (2020). TF-IDF vs word embeddings for
morbidity identification in clinical notes: An initial
study. In Consoli, S., Recupero, D. R., and Ri-
boni, D., editors, Proceedings of the First Workshop
on Smart Personal Health Interfaces co-located with
25th International Conference on Intelligent User In-
terfaces, SmartPhil@IUI 2020, Cagliari, Italy, March
17, 2020, volume 2596 of CEUR Workshop Proceed-
ings, pages 1–12. CEUR-WS.org.
Dhole, K. D., Gangal, V., Gehrmann, S., Gupta, A., Li, Z.,
Mahamood, S., Mahendiran, A., Mille, S., Srivastava,
A., Tan, S., et al. (2021). Nl-augmenter: A frame-
work for task-sensitive natural language augmenta-
tion. arXiv preprint arXiv:2112.02721.
Flemotomos, N., Martinez, V. R., Chen, Z., Singla, K.,
Ardulov, V., Peri, R., Caperton, D. D., Gibson, J.,
Tanana, M. J., Georgiou, P., et al. (2022). Automated
evaluation of psychotherapy skills using speech and
language technologies. Behavior Research Methods,
54(2):690–711.
Gibson, J., Can, D., Xiao, B., Imel, Z. E., Atkins, D. C.,
Georgiou, P., and Narayanan, S. S. (2016). A Deep
Learning Approach to Modeling Empathy in Addic-
tion Counseling. In Proc. Interspeech 2016, pages
1447–1451.
Gibson, J., Malandrakis, N., Romero, F., Atkins, D. C., and
Narayanan, S. S. (2015). Predicting therapist empathy
in motivational interviews using language features in-
spired by psycholinguistic norms. In Sixteenth annual
conference of the international speech communication
association.
Ibrahim, H., Liu, X., Zariffa, N., Morris, A. D., and Den-
niston, A. K. (2021). Health data poverty: an assail-
able barrier to equitable digital health care. The Lancet
Digital Health, 3(4):e260–e265.
John-Mathews, J.-M., Cardon, D., and Balagu
´
e, C. (2022).
From reality to world. a critical perspective on ai fair-
ness. Journal of Business Ethics, pages 1–15.
Kumar, V., Mishra, B. K., Mazzara, M., Thanh, D. N., and
Verma, A. (2020a). Prediction of malignant and be-
nign breast cancer: A data mining approach in health-
care applications. In Advances in data science and
management, pages 435–442. Springer.
Kumar, V., Recupero, D. R., Riboni, D., and Helaoui, R.
(2020b). Ensembling classical machine learning and
deep learning approaches for morbidity identification
from clinical notes. IEEE Access, 9:7107–7126.
Le Glaz, A., Haralambous, Y., Kim-Dufor, D.-H., Lenca, P.,
Billot, R., Ryan, T. C., Marsh, J., Devylder, J., Wal-
ter, M., Berrouiguet, S., et al. (2021). Machine learn-
ing and natural language processing in mental health:
Systematic review. Journal of Medical Internet Re-
search, 23(5):e15708.
Li, B., Hou, Y., and Che, W. (2022). Data augmentation
approaches in natural language processing: A survey.
AI Open.
Locke, S., Bashall, A., Al-Adely, S., Moore, J., Wilson, A.,
and Kitchen, G. B. (2021). Natural language process-
ing in medicine: a review. Trends in Anaesthesia and
Critical Care, 38:4–9.
Miller, W. R. and Rollnick, S. (2012). Motivational inter-
viewing: Helping people change. Guilford press.
P
´
erez-Rosas, V., Wu, X., Resnicow, K., and Mihalcea, R.
(2019). What makes a good counselor? learning
to distinguish between high-quality and low-quality
counseling conversations. In Proceedings of the 57th
Annual Meeting of the Association for Computational
Linguistics, pages 926–935, Florence, Italy. Associa-
tion for Computational Linguistics.
Rice, M. E. and Harris, G. T. (2005). Comparing effect sizes
in follow-up studies: Roc area, cohen’s d, and r. Law
and human behavior, 29(5):615–620.
Rollnick, S., Miller, W. R., and Butler, C. (2008). Moti-
vational interviewing in health care: helping patients
change behavior. Guilford Press.
Wu, Z., Balloccu, S., Kumar, V., Helaoui, R., Reiter, E.,
Recupero, D. R., and Riboni, D. (2022). Anno-
mi: A dataset of expert-annotated counselling dia-
logues. In ICASSP 2022-2022 IEEE International
Conference on Acoustics, Speech and Signal Process-
ing (ICASSP), pages 6177–6181. IEEE.
Wu, Z., Helaoui, R., Kumar, V., Reforgiato Recupero,
D., and Riboni, D. (2020). Towards detecting need
for empathetic response in motivational interviewing.
In Companion Publication of the 2020 International
Conference on Multimodal Interaction, pages 497–
502.
Xiao, B., Can, D., Georgiou, P. G., Atkins, D., and
Narayanan, S. S. (2012). Analyzing the language
of therapist empathy in motivational interview based
psychotherapy. In Proceedings of The 2012 Asia Pa-
cific Signal and Information Processing Association
Annual Summit and Conference, pages 1–4. IEEE.
Xiao, B., Can, D., Gibson, J., Imel, Z. E., Atkins, D. C.,
Georgiou, P. G., and Narayanan, S. S. (2016). Behav-
ioral coding of therapist language in addiction coun-
seling using recurrent neural networks. In Interspeech,
pages 908–912.
SDAIH 2022 - Scarce Data in Artificial Intelligence for Healthcare
28