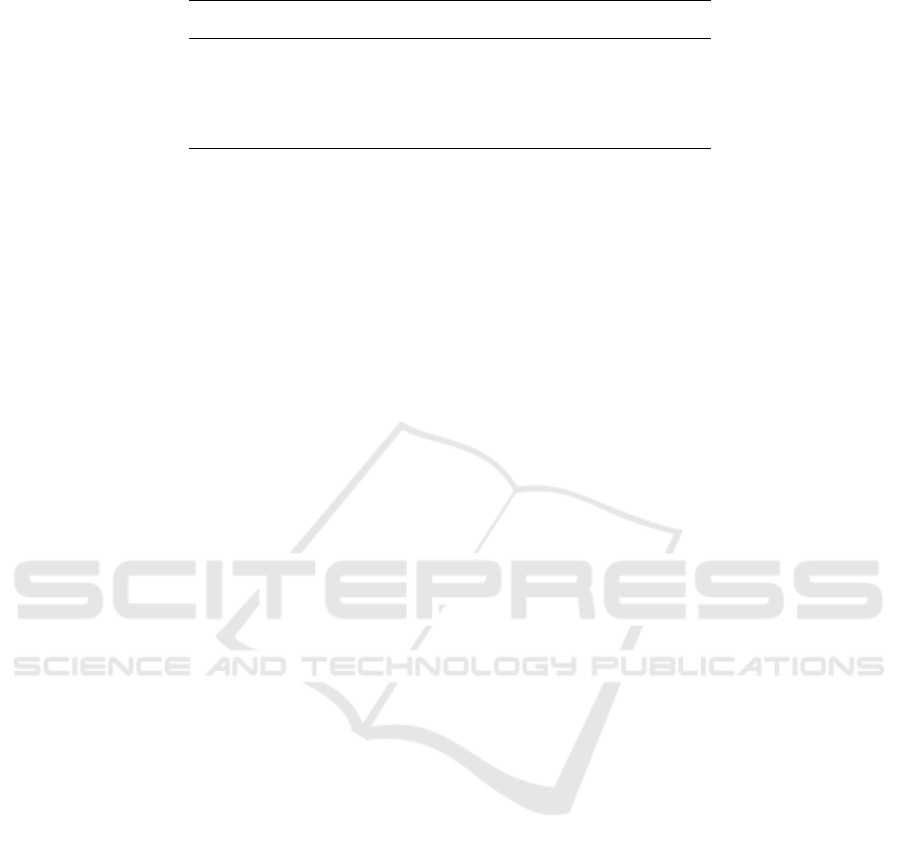
Table 4: Users that found the privacy policy easier to understand per age group.
Age group # users Strongly Agree Neutral Disagree Strongly
Agree Disagree
18-24 36 52.8% 33.3% 11.1% 2.8% 0%
25-29 17 29.4% 47.1% 17.6% 5.9% 0%
30-39 8 12.5% 50% 25% 0% 12.5%
40-49 13 7.7% 69.2% 23.1% 0% 0%
50-59 13 30.8% 38.5% 30.8% 0% 0%
¿60 2 0% 0% 100% 0% 0%
ing dataset, may have lead to a lower accuracy
in the results.
The classifier shows lower accuracy than Poli-
sis (Harkous et al., 2018), which has an average accu-
racy of 88.4% but it is on the same level as other ap-
proaches such as PrivacyCheck (Zaeem et al., 2018),
which has an accuracy of 40%-73%. Future work
will examine whether the use of unsupervised tech-
niques or a combination of supervised and unsuper-
vised techniques used in previous works can improve
the accuracy (Harkous et al., 2018; Sarne et al., 2019).
Regarding the user study, it is affected by external
validity, referring to the extent we can generalize our
findings. Although our user sample included users of
various backgrounds (i.e. ages and expertise), a larger
sample may provide slightly different observations.
7 CONCLUSIONS
In this paper, we have presented our work on more
user friendly representations of the text of privacy
policies via Privacy Policy Beautifier, where policies
are presented to users in different ways: textual with
text highlighting, in the form of a pie chart, as a
word cloud and as a table with indications of the pres-
ence of GDPR terms. The classification accuracy of
the proposed classifier shows promising results (74%)
that can be further improved, whereas the user study
showed that users value the different representations
with many users having a positive interaction with
different representations. Future work will examine
whether the use of unsupervised techniques or a com-
bination of supervised and unsupervised techniques
can improve the classification accuracy. As part of
future work, we also intend to enhance Privacy Pol-
icy Beautifier by adding a summarization of the text,
and by considering the addition of more representa-
tions that have been studied in previous works, while
studying their effect on the user experience (Soumeli-
dou and Tsohou, 2019).
REFERENCES
Angulo, J., Fischer-H
¨
ubner, S., W
¨
astlund, E., and Pulls, T.
(2012). Towards usable privacy policy display and
management. Information Management & Computer
Security.
Dhar, A., Mukherjee, H., Dash, N. S., and Roy, K. (2021).
Text categorization: past and present. Artificial Intel-
ligence Review, 54(4):3007–3054.
Gall
´
e, M., Christofi, A., and Elsahar, H. (2019). The case
for a gdpr-specific annotated dataset of privacy poli-
cies. In AAAI Symposium on Privacy-Enhancing AI
and HLT Technologies.
Gardner, M. W. and Dorling, S. (1998). Artificial neural
networks (the multilayer perceptron)—a review of ap-
plications in the atmospheric sciences. Atmospheric
environment, 32(14-15):2627–2636.
Harkous, H., Fawaz, K., Lebret, R., Schaub, F., Shin,
K. G., and Aberer, K. (2018). Polisis: Automated
analysis and presentation of privacy policies using
deep learning. In 27th {USENIX} security symposium
({USENIX} security 18), pages 531–548.
Kelley, P. G., Bresee, J., Cranor, L. F., and Reeder, R. W.
(2009). A” nutrition label” for privacy. In Proceedings
of the 5th Symposium on Usable Privacy and Security,
pages 1–12.
Kim, K., Ko, S., Elmqvist, N., and Ebert, D. S. (2011).
Wordbridge: Using composite tag clouds in node-link
diagrams for visualizing content and relations in text
corpora. In 2011 44th Hawaii International Confer-
ence on System Sciences, pages 1–8. IEEE.
Kumar, V. B., Ravichander, A., Story, P., and Sadeh, N.
(2019). Quantifying the effect of in-domain dis-
tributed word representations: A study of privacy
policies. In AAAI Spring Symposium on Privacy-
Enhancing Artificial Intelligence and Language Tech-
nologies.
Lebanoff, L. and Liu, F. (2018). Automatic detection of
vague words and sentences in privacy policies. arXiv
preprint arXiv:1808.06219.
Leung, K. M. (2007). Naive bayesian classifier. Polytechnic
University Department of Computer Science/Finance
and Risk Engineering, 2007:123–156.
Liaw, A., Wiener, M., et al. (2002). Classification and re-
gression by randomforest. R news, 2(3):18–22.
Linden, T., Khandelwal, R., Harkous, H., and Fawaz, K.
(2018). The privacy policy landscape after the gdpr.
arXiv preprint arXiv:1809.08396.
WEBIST 2022 - 18th International Conference on Web Information Systems and Technologies
62