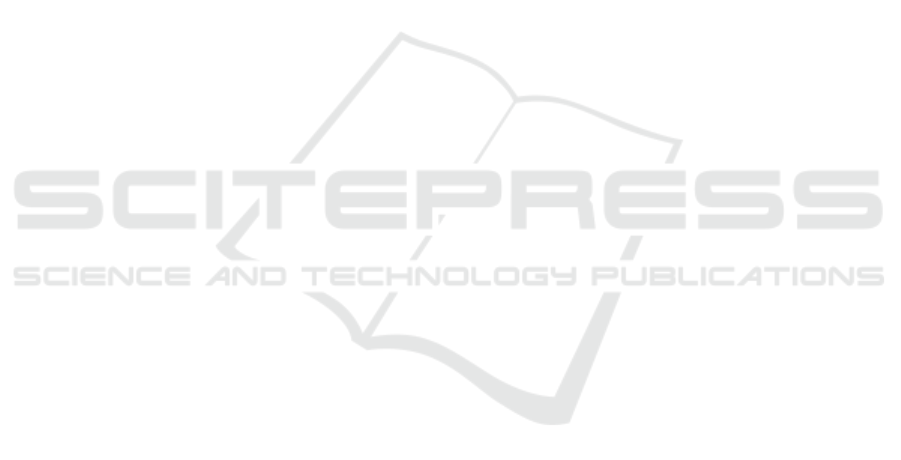
being the most interesting candidates. This was con-
firmed by a rigorous statistical analysis. Interestingly,
we found that where ES can be expected to perform
better on error than NSLC, it can also be expected to
yield larger solution sizes (a higher number of rules
in the model) and vice versa.
Overall, we recommend to use either ES or NSLC,
although, due to its greater simplicity and the fact that
rules are selected independently of the status of other
rules, ES seems to be the preferential candidate for
cases where model construction is important for the
explainability requirements of users.
ACKNOWLEDGEMENTS
This work was partially funded by the Bavarian Min-
istry of Economic Affairs, Energy and Technology.
REFERENCES
Akiba, T., Sano, S., Yanase, T., Ohta, T., and Koyama,
M. (2019). Optuna: A Next-generation Hyperpa-
rameter Optimization Framework. In Proceedings
of the 25th ACM SIGKDD International Conference
on Knowledge Discovery & Data Mining, KDD ’19,
pages 2623–2631, New York, NY, USA. Association
for Computing Machinery.
Benavoli, A., Corani, G., Dem
ˇ
sar, J., and Zaffalon, M.
(2017). Time for a change: A tutorial for compar-
ing multiple classifiers through bayesian analysis. J.
Mach. Learn. Res., 18(1):2653–2688.
Brooks, T., Pope, D., and Marcolini, M. (1989). Airfoil
Self-Noise and Prediction. Technical Report RP-1218,
NASA.
Calvo, B., Ceberio, J., and Lozano, J. A. (2018). Bayesian
inference for algorithm ranking analysis. In Proceed-
ings of the Genetic and Evolutionary Computation
Conference Companion, GECCO ’18, pages 324–325,
New York, NY, USA. Association for Computing Ma-
chinery.
Calvo, B., Shir, O. M., Ceberio, J., Doerr, C., Wang, H.,
B
¨
ack, T., and Lozano, J. A. (2019). Bayesian per-
formance analysis for black-box optimization bench-
marking. In Proceedings of the Genetic and
Evolutionary Computation Conference Companion,
GECCO ’19, pages 1789–1797, New York, NY, USA.
Association for Computing Machinery.
Corani, G. and Benavoli, A. (2015). A bayesian approach
for comparing cross-validated algorithms on multiple
data sets. Machine Learning, 100(2):285–304.
Dua, D. and Graff, C. (2017). UCI machine learning repos-
itory. http://archive.ics.uci.edu/ml.
Gomes, J., Mariano, P., and Christensen, A. L. (2015). De-
vising effective novelty search algorithms: A com-
prehensive empirical study. In Proceedings of the
2015 Annual Conference on Genetic and Evolution-
ary Computation, GECCO ’15, page 943–950, New
York, NY, USA. Association for Computing Machin-
ery.
Gomes, J., Urbano, P., and Christensen, A. L. (2012). Pro-
gressive minimal criteria novelty search. In Pav
´
on,
J., Duque-M
´
endez, N. D., and Fuentes-Fern
´
andez,
R., editors, Advances in Artificial Intelligence – IB-
ERAMIA 2012, pages 281–290. Springer Berlin Hei-
delberg.
Heider, M., Nordsieck, R., and H
¨
ahner, J. (2021). Learn-
ing Classifier Systems for Self-Explaining Socio-
Technical-Systems. In Stein, A., Tomforde, S., Botev,
J., and Lewis, P., editors, Proceedings of LIFELIKE
2021 co-located with 2021 Conference on Artificial
Life (ALIFE 2021).
Heider, M., Stegherr, H., Nordsieck, R., and H
¨
ahner,
J. (2022a). Learning classifier systems for self-
explaining socio-technical-systems. https://arxiv.org/
abs/2207.02300. Submitted as an extended version of
(Heider et al., 2021).
Heider, M., Stegherr, H., Wurth, J., Sraj, R., and H
¨
ahner, J.
(2022b). Separating Rule Discovery and Global So-
lution Composition in a Learning Classifier System.
In Genetic and Evolutionary Computation Conference
Companion (GECCO ’22 Companion).
Heider, M., Stegherr, H., Wurth, J., Sraj, R., and H
¨
ahner, J.
(2022c). Investigating the impact of independent rule
fitnesses in a learning classifier system. http://arxiv.
org/abs/2207.05582. Accepted for publication in the
Proceedings of BIOMA’22.
Kaya, H. and T
¨
ufekci, P. (2012). Local and Global Learn-
ing Methods for Predicting Power of a Combined Gas
& Steam Turbine. In Proceedings of the Interna-
tional Conference on Emerging Trends in Computer
and Electronics Engineering ICETCEE.
Lehman, J. (2012). Evolution Through the Search for Nov-
elty. PhD thesis, University of Central Florida.
Lehman, J. and Stanley, K. O. (2010). Revising the evo-
lutionary computation abstraction: Minimal criteria
novelty search. In Proceedings of the 12th Annual
Conference on Genetic and Evolutionary Computa-
tion, GECCO ’10, page 103–110, New York, NY,
USA. Association for Computing Machinery.
Pedregosa, F., Varoquaux, G., Gramfort, A., Michel, V.,
Thirion, B., Grisel, O., Blondel, M., Prettenhofer, P.,
Weiss, R., Dubourg, V., Vanderplas, J., Passos, A.,
Cournapeau, D., Brucher, M., Perrot, M., and Duch-
esnay,
´
E. (2011). Scikit-learn: Machine Learning in
Python. The Journal of Machine Learning Research,
12:2825–2830.
Tsanas, A. and Xifara, A. (2012). Accurate Quantitative Es-
timation of Energy Performance of Residential Build-
ings Using Statistical Machine Learning Tools. En-
ergy and Buildings, 49:560–567.
T
¨
ufekci, P. (2014). Prediction of full load electrical power
output of a base load operated combined cycle power
plant using machine learning methods. Interna-
tional Journal of Electrical Power & Energy Systems,
60:126–140.
ECTA 2022 - 14th International Conference on Evolutionary Computation Theory and Applications
48