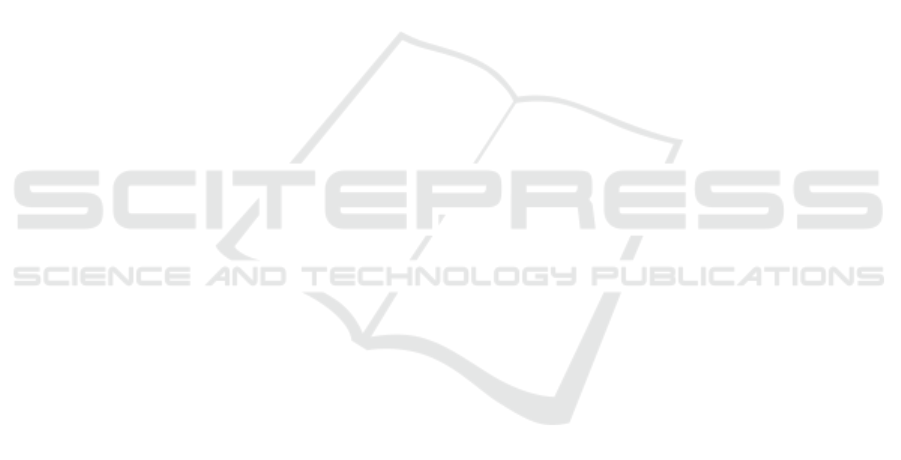
pert and casual users. Lecture Notes in Computer Sci-
ence, pages 274–286, Berlin, Heidelberg. Springer.
Fox, E. A. (2020). How should one explore the digital
library of the future? JCDL ’20, page 1–2, New
York, NY, USA. Association for Computing Machin-
ery. [Online; accessed 2021-05-16].
Hassan Awadallah, A., White, R. W., Pantel, P., Dumais,
S. T., and Wang, Y.-M. (2014). Supporting complex
search tasks. In Proceedings of the 23rd ACM interna-
tional conference on information and knowledge man-
agement, pages 829–838.
Hu, X., Duan, J., and Dang, D. (2021). Natural language
question answering over knowledge graph: the mar-
riage of sparql query and keyword search. Knowledge
and Information Systems, 63(4):819–844.
Jayaram, N., Khan, A., Li, C., Yan, X., and Elmasri,
R. (2014). Towards a query-by-example system for
knowledge graphs. GRADES’14, page 1–6, New
York, NY, USA. Association for Computing Machin-
ery. [Online; accessed 2021-04-12].
Jayaram, N., Khan, A., Li, C., Yan, X., and Elmasri, R.
(2015). Querying knowledge graphs by example en-
tity tuples. IEEE Transactions on Knowledge and
Data Engineering, 27(10):2797–2811. event: IEEE
Transactions on Knowledge and Data Engineering.
Kuric, E., Fern
´
andez, J. D., and Drozd, O. (2019). Knowl-
edge graph exploration: A usability evaluation of
query builders for laypeople. Lecture Notes in Com-
puter Science, pages 326–342, Cham. Springer Inter-
national Publishing.
Lazonder, A. W., Biemans, H. J., and Wopereis, I. G.
(2000). Differences between novice and experienced
users in searching information on the world wide web.
Journal of the American Society for Information Sci-
ence, 51(6):576–581.
Liang, S., Stockinger, K., de Farias, T. M., Anisimova, M.,
and Gil, M. (2021). Querying knowledge graphs in
natural language. Journal of Big Data, 8(1):3.
Lissandrini, M., Mottin, D., Palpanas, T., and Velegrakis,
Y. (2020). Graph-query suggestions for knowledge
graph exploration. WWW ’20, page 2549–2555, New
York, NY, USA. Association for Computing Machin-
ery. [Online; accessed 2021-04-12].
Mohanty, M. and Ramanath, M. (2019). Insta-search:
Towards effective exploration of knowledge graphs.
CIKM ’19, page 2909–2912, New York, NY, USA.
Association for Computing Machinery. [Online; ac-
cessed 2021-04-12].
Namaki, M. H., Wu, Y., and Zhang, X. (2018). Gexp: Cost-
aware graph exploration with keywords. SIGMOD
’18, page 1729–1732, New York, NY, USA. Associ-
ation for Computing Machinery. [Online; accessed
2021-04-12].
Norman, D. A. (2002). The design of everyday things. Basic
Books, New York, 1st basic paperback edition.
Pirolli, P. (2009). Powers of 10: Modeling complex
information-seeking systems at multiple scales. Com-
puter, 42(3):33–40. event: Computer.
Riesen, K., Witschel, H.-F., and Grether, L. (2021). A novel
data set for information retrieval on the basis of sub-
graph matching. In Torsello, A., Rossi, L., Pelillo,
M., Biggio, B., and Robles-Kelly, A., editors, Struc-
tural, Syntactic, and Statistical Pattern Recognition,
volume 12644, pages 205–215. Springer International
Publishing, Cham.
Rogers, Y., Scaife, M., and Navarro-Prieto, R. (1999). Cog-
nitive strategies in web searching. Proceedings of the
5th . . . . [Online; accessed 2021-11-09].
R
´
asto
ˇ
cn
´
y, K., Tvaro
ˇ
zek, M., and Bielikov
´
a, M. (2011).
Supporting search result browsing and exploration via
cluster-based views and zoom-based navigation. WI-
IAT ’11, page 297–300, USA. IEEE Computer Soci-
ety. [Online; accessed 2021-04-12].
Tabatabai, D. and Shore, B. (2005). How experts and
novices search the web. Library & Information Sci-
ence Research, 27:222–248.
Tominski, C., Abello, J., and Schumann, H. (2009).
Cgv—an interactive graph visualization system. Com-
puters & Graphics, 33(6):660–678.
White, R. W., Dumais, S. T., and Teevan, J. (2009). Char-
acterizing the influence of domain expertise on web
search behavior. WSDM ’09, page 132–141, New
York, NY, USA. Association for Computing Machin-
ery. [Online; accessed 2021-11-02].
Witschel, H. F., Riesen, K., and Grether, L. (2020). Kvgr: A
graph-based interface for explorative sequential ques-
tion answering on heterogeneous information sources.
Lecture Notes in Computer Science, pages 760–773,
Cham. Springer International Publishing.
Witschel, H. F., Riesen, K., and Grether, L. (2021). Natural
language-based user guidance for knowledge graph
exploration: A user study. In Cucchiara, R., Fred, A.
L. N., and Filipe, J., editors, Proceedings of the 13th
International Joint Conference on Knowledge Discov-
ery, Knowledge Engineering and Knowledge Manage-
ment, IC3K 2021, Volume 1: KDIR, Online Streaming,
October 25-27, 2021, pages 95–102. SCITEPRESS.
Wu, Y., Yang, S., Srivatsa, M., Iyengar, A., and Yan, X.
(2013). Summarizing answer graphs induced by key-
word queries. Proceedings of the VLDB Endowment,
6(14):1774–1785.
Yahya, M. (2016). Question answering and query process-
ing for extended knowledge graphs. page 172.
Yang, S., Xie, Y., Wu, Y., Wu, T., Sun, H., Wu, J., and
Yan, X. (2014). Slq: a user-friendly graph querying
system. pages 893–896, Snowbird Utah USA. SIG-
MOD/PODS’14: International Conference on Man-
agement of Data, ACM. [Online; accessed 2021-03-
09].
Yi, P., Choi, B., Bhowmick, S. S., and Xu, J. (2017). Autog:
a visual query autocompletion framework for graph
databases. The VLDB Journal — The International
Journal on Very Large Data Bases, 26(3):347–372.
Zafar, H., Dubey, M., Lehmann, J., and Demidova, E.
(2020). Iqa: Interactive query construction in seman-
tic question answering systems. Journal of Web Se-
mantics, 64.
KDIR 2022 - 14th International Conference on Knowledge Discovery and Information Retrieval
264