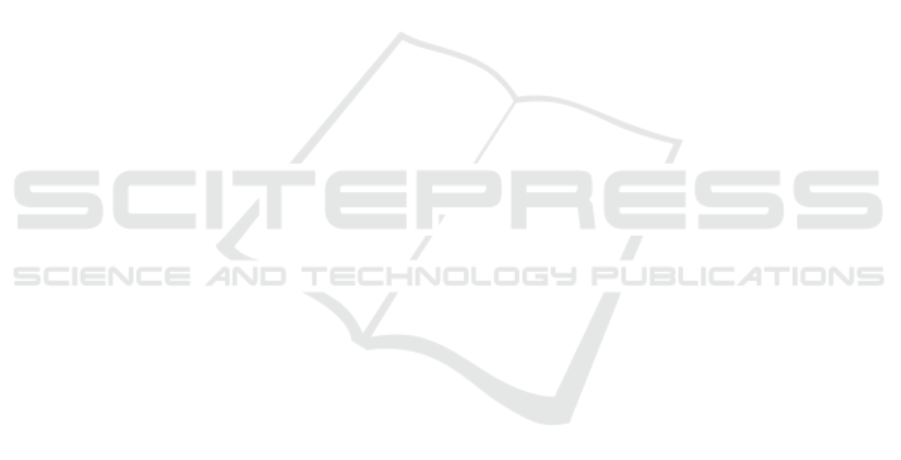
Duan, N., Tang, D., Chen, P., and Zhou, M. (2017).
Question generation for question answering. In Pro-
ceedings of the 2017 Conference on Empirical Meth-
ods in Natural Language Processing, pages 866–
874, Copenhagen, Denmark. Association for Compu-
tational Linguistics.
Flor, M. and Riordan, B. (2018). A semantic role-based
approach to open-domain automatic question gener-
ation. In Proceedings of the thirteenth workshop on
innovative use of NLP for building educational appli-
cations, pages 254–263.
Harrison, V. and Walker, M. (2018). Neural generation
of diverse questions using answer focus, contextual
and linguistic features. Association for Computational
Linguistics.
Khullar, P., Rachna, K., Hase, M., and Shrivastava, M.
(2018). Automatic question generation using relative
pronouns and adverbs. In Proceedings of ACL 2018,
Student Research Workshop, pages 153–158.
Kim, Y., Lee, H., Shin, J., and Jung, K. (2019). Improv-
ing neural question generation using answer separa-
tion. volume 33, page 6602–6609. Association for the
Advancement of Artificial Intelligence (AAAI).
Lavie, A. and Denkowski, M. J. (2009). The meteor metric
for automatic evaluation of machine translation. Ma-
chine Translation, 23(2—3):105–115.
Lin, C.-Y. (2004). ROUGE: a package for automatic evalu-
ation of summaries. In Text Summarization Branches
Out, pages 74–81, Barcelona, Spain. Association for
Computational Linguistics.
Lindberg, D., Popowich, F., Nesbit, J., and Winne, P.
(2013). Generating natural language questions to sup-
port learning on-line. In Proceedings of the 14th Eu-
ropean Workshop on Natural Language Generation,
pages 105–114.
Lindberg, D. L. (2013). Automatic question generation from
text for self-directed learning. PhD thesis, Applied
Sciences: School of Computing Science.
Luong, T., Pham, H., and Manning, C. D. (2015). Ef-
fective approaches to attention-based neural machine
translation. In Proceedings of the 2015 Conference on
Empirical Methods in Natural Language Processing,
pages 1412–1421, Lisbon, Portugal. Association for
Computational Linguistics.
Mannem, P., Prasad, R., and Joshi, A. (2010). Question
generation from paragraphs at upenn: Qgstec system
description. In Proceedings of QG2010: The Third
Workshop on Question Generation, pages 84–91.
Marcus, M., Santorini, B., and Marcinkiewicz, M. A.
(1993). Building a large annotated corpus of english:
The penn treebank.
Martha, P., Dan, G., and Paul, K. (2005). The proposition
bank: a corpus annotated with semantic roles. Com-
putational Linguistics Journal, 31(1):10–1162.
Mazidi, K. and Nielsen, R. (2014). Linguistic considera-
tions in automatic question generation. In Proceed-
ings of the 52nd Annual Meeting of the Association for
Computational Linguistics (Volume 2: Short Papers),
pages 321–326.
Mostow, J. and Chen, W. (2009). Generating instruc-
tion automatically for the reading strategy of self-
questioning. In AIED, pages 465–472.
Papineni, K., Roukos, S., Ward, T., and Zhu, W.-J. (2002).
BLEU: a method for automatic evaluation of ma-
chine translation. In Proceedings of the 40th Annual
Meeting on Association for Computational Linguis-
tics, ACL’02, page 311–318, USA. Association for
Computational Linguistics.
Peters, M. E., Ammar, W., Bhagavatula, C., and Power,
R. (2017). Semi-supervised sequence tagging with
bidirectional language models. arXiv preprint
arXiv:1705.00108.
Raffel, C., Shazeer, N., Roberts, A., Lee, K., Narang, S.,
Matena, M., Zhou, Y., Li, W., and Liu, P. J. (2020).
Exploring the limits of transfer learning with a unified
text-to-text transformer.
Rajpurkar, P., Zhang, J., Lopyrev, K., and Liang, P. (2016).
Squad: 100,000+ questions for machine comprehen-
sion of text. In Proceedings of the 2016 Conference
on Empirical Methods in Natural Language Process-
ing, pages 2383–2392. Association for Computational
Linguistics.
Rus, V., Cai, Z., and Graesser, A. C. (2007). Experiments
on generating questions about facts. In Proceedings of
the International Conference on Intelligent Text Pro-
cessing and Computational Linguistics, pages 444–
455. Springer.
Sachan, M. and Xing, E. (2018). Self-training for jointly
learning to ask and nnswer questions. In Proceedings
of the 2018 Conference of the North American Chap-
ter of the Association for Computational Linguistics:
Human Language Technologies, Volume 1 (Long Pa-
pers), pages 629–640, New Orleans, Louisiana. Asso-
ciation for Computational Linguistics.
Sak, H., Senior, A. W., and Beaufays, F. (2014). Long
short-term memory recurrent neural network architec-
tures for large scale acoustic modeling. In Procced-
ings of the 15th Annual Conference of the Interna-
tional Speech Communication Association.
Shi, P. and Lin, J. (2019). Simple BERT models for relation
extraction and semantic role labeling. arXiv preprint
arXiv:1904.05255.
Song, L., Wang, Z., Hamza, W., Zhang, Y., and Gildea,
D. (2018). Leveraging context information for natural
question generation. In Proceedings of the 2018 Con-
ference of the North American Chapter of the Associa-
tion for Computational Linguistics: Human Language
Technologies, Volume 2 (Short Papers), pages 569–
574, New Orleans, Louisiana. Association for Com-
putational Linguistics.
Toutanova, K., Klein, D., Manning, C. D., and Singer, Y.
(2003). Feature-rich part-of-speech tagging with a
cyclic dependency network. In Proceedings of the
2003 conference of the North American chapter of
the association for computational linguistics on hu-
man language technology-volume 1, pages 173–180.
Association for Computational Linguistics.
Ukkonen, E. (1985). Algorithms for approximate string
matching. Information and control, 64(1-3):100–118.
KDIR 2022 - 14th International Conference on Knowledge Discovery and Information Retrieval
146