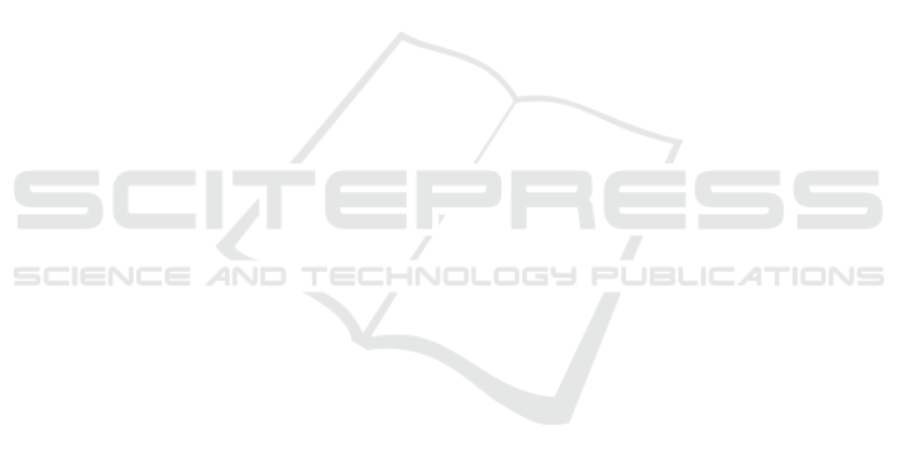
sidering the given use case. As a future work, we
aim to deepen the problem of anomalies produced
by air quality monitoring sensors. Such sensors are
more sensitive to environmental changes than traf-
fic sensors, their observations are strongly affected
by the values of humidity, temperature and also the
concentrations of other gases or particles (Rollo and
Po, 2021; Rollo et al., 2021; Bachechi et al., 2020b).
Moreover, air quality sensors are subject to rapid de-
terioration over time.
ACKNOWLEDGEMENTS
Research reported in this paper was partially sup-
ported by the TRAFAIR project 2017-EU-IA-0167,
co-financed by the Connecting Europe Facility of the
European Union. The views and conclusions con-
tained in this document are those of the authors and
should not be interpreted as representing the official
policies, either expressed or implied, of the EU Com-
mission. The authors would like to thank in particular
the partners that contribute to the collection and man-
agement of traffic sensor data: the City of Modena
and Lepida S.c.p.A..
REFERENCES
Ali, K., Anwar, T., Naqvi, I. H., and Jafry, M. H. (2015).
Composite event detection and identification for wsns
using general hebbian algorithm. In 2015 IEEE In-
ternational Conference on Communications (ICC),
pages 6463–6468.
Bachechi, C., Desimoni, F., Po, L., and Casas, D. M.
(2020a). Visual analytics for spatio-temporal air qual-
ity data. In 2020 24th International Conference Infor-
mation Visualisation (IV), pages 460–466.
Bachechi, C., Desimoni, F., Po, L., and Casas, D. M.
(2020b). Visual analytics for spatio-temporal air qual-
ity data. In Banissi, E., Khosrow-shahi, F., Ursyn,
A., Bannatyne, M. W. M., Pires, J. M., Datia, N.,
Nazemi, K., Kovalerchuk, B., Counsell, J., Agapiou,
A., Vrcelj, Z., Chau, H., Li, M., Nagy, G., Laing, R.,
Francese, R., Sarfraz, M., Bouali, F., Venturini, G.,
Trutschl, M., Cvek, U., M
¨
uller, H., Nakayama, M.,
Temperini, M., Mascio, T. D., Sciarrone, F., Rossano,
V., D
¨
orner, R., Caruccio, L., Vitiello, A., Huang,
W., Risi, M., Erra, U., Andonie, R., Ahmad, M. A.,
Figueiras, A., Cuzzocrea, A., and Mabakane, M. S.,
editors, 24th International Conference on Information
Visualisation, IV 2020, Melbourne, Australia, Septem-
ber 7-11, 2020, pages 460–466. IEEE.
Bachechi, C. and Po, L. (2019). Implementing an urban
dynamic traffic model. In Barnaghi, P. M., Gottlob,
G., Manolopoulos, Y., Tzouramanis, T., and Vakali,
A., editors, 2019 IEEE/WIC/ACM International Con-
ference on Web Intelligence, WI 2019, Thessaloniki,
Greece, October 14-17, 2019, pages 312–316. ACM.
Bachechi, C., Po, L., and Rollo, F. (2022a). Big data ana-
lytics and visualization in traffic monitoring. Big Data
Res., 27:100292.
Bachechi, C., Rollo, F., Desimoni, F., and Po, L. (2020c).
Using real sensors data to calibrate a traffic model for
the city of modena. In Ahram, T. Z., Karwowski,
W., Vergnano, A., Leali, F., and Ta
¨
ıar, R., editors, In-
telligent Human Systems Integration 2020 - Proceed-
ings of the 3rd International Conference on Intelligent
Human Systems Integration (IHSI 2020): Integrating
People and Intelligent Systems, February 19-21, 2020,
Modena, Italy, volume 1131 of Advances in Intelligent
Systems and Computing, pages 468–473. Springer.
Bachechi, C., Rollo, F., and Po, L. (2020d). Real-time data
cleaning in traffic sensor networks. In 17th IEEE/ACS
International Conference on Computer Systems and
Applications, AICCSA 2020, Antalya, Turkey, Novem-
ber 2-5, 2020, pages 1–8. IEEE.
Bachechi, C., Rollo, F., and Po, L. (2022b). Detection and
classification of sensor anomalies for simulating urban
traffic scenarios. Clust. Comput., 25(4):2793–2817.
Bachechi, C., Rollo, F., Po, L., and Quattrini, F. (2021).
Anomaly detection in multivariate spatial time series:
A ready-to-use implementation. In Mayo, F. J. D.,
Marchiori, M., and Filipe, J., editors, Proceedings of
the 17th International Conference on Web Informa-
tion Systems and Technologies, WEBIST 2021, Octo-
ber 26-28, 2021, pages 509–517. SCITEPRESS.
Bianco, A., Ben, M. G., Mart
´
ınez, E., and Yohai, V. (2001).
Outlier detection in regression models with arima er-
rors using robust estimates. J. Forecast., 20:565–579.
Chander, B. and Kumaravelan, G. (2022). Outlier de-
tection strategies for wsns: A survey. Journal of
King Saud University - Computer and Information
Sciences, 34(8):5684–5707. Cited By :3.
Chandola, V., Mithal, V., and Kumar, V. (2008). Compar-
ative evaluation of anomaly detection techniques for
sequence data. In Proceedings of the 8th IEEE Inter-
national Conference on Data Mining (ICDM 2008),
pages 743–748.
Desimoni, F., Ilarri, S., Po, L., Rollo, F., and Trillo-Lado,
R. (2020). Semantic traffic sensor data: The trafair
experience. Applied Sciences, 10(17).
G
¨
ornitz, N., Kloft, M., Rieck, K., and Brefeld, U. (2012).
Toward supervised anomaly detection. Journal of Ar-
tificial Intelligence Research (JAIR), 45.
Kurian, N., Thomas, A., and George, B. (2015). Automated
fault diagnosis in multiple inductive loop detectors.
11th IEEE India Conference: Emerging Trends and
Innovation in Technology, INDICON 2014.
Moshtaghi, M., Leckie, C., Karunasekera, S., Bezdek, J. C.,
Rajasegarar, S., and Palaniswami, M. (2011). Incre-
mental elliptical boundary estimation for anomaly de-
tection in wireless sensor networks. In Cook, D. J.,
Pei, J., Wang, W., Za
¨
ıane, O. R., and Wu, X., editors,
11th IEEE International Conference on Data Mining,
ICDM 2011, Vancouver, BC, Canada, December 11-
14, 2011, pages 467–476. IEEE Computer Society.
Semi Real-time Data Cleaning of Spatially Correlated Data in Traffic Sensor Networks
93