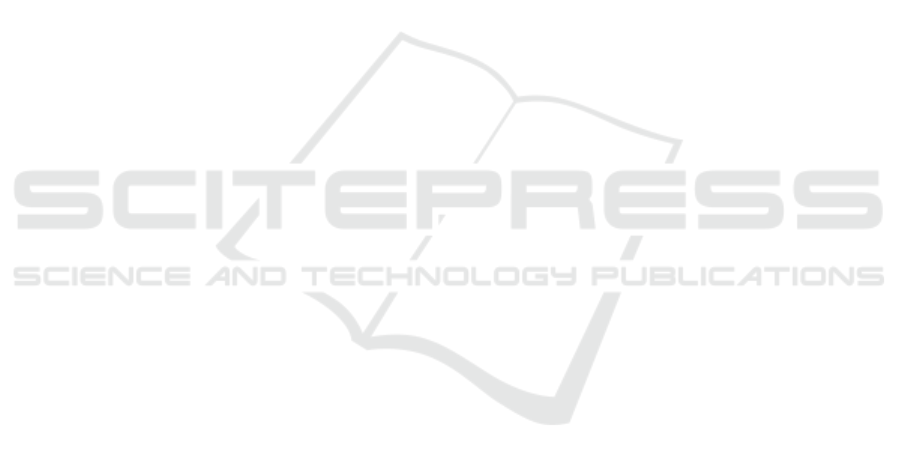
mutually contradictory evidence regarding the impact
of intangible assets on the financial performance. In
general, this does not allow the management of enter-
prises to effectively control intangible values aimed
at creating internal value, and for investors – to re-
ceive clear signals for making effective investments.
Considering the above, the following objectives were
formulated: to measure the relationship between in-
tangible assets and the financial performance of Slo-
vak ICT companies; to investigate which components
of intangible assets have the most significant or in-
significant impact on the financial performance of
Slovak ICT companies; to form recommendations for
improving the investment policy of ICT companies,
based on the level of significance of the elements of
intangible assets from the point of view of increasing
financial results.
3 DATA AND METHODOLOGY
Sample selection. To determine whether intangible
assets stimulate financial performance, was analyzed
sample of 180 Slovak ICT companies for the period
2015–2019. In particular, the panel data information
from financial statements of such enterprises, avail-
able in the open access, as well as the information
from database “FinStat” was used to form panel data.
Only those companies, for which the necessary infor-
mation for the 5-year period was available, were in-
cluded in the sample. The selected 180 companies
provide a valid and complete set of data in order to
carry out relevant statistical analysis.
Investigated enterprises proceeding from EU Eco-
nomic Activity Classification and from the SK NACE
2 classification belongs to group 26 “Manufacture
of computer, electronic and optical products”, in-
cludes direct production of computers, computer pe-
ripheral equipment (input device, output device, in-
put/output device), communication equipment (public
switching equipment, transmission equipment, cus-
tomer premises equipment), measuring, medical, nav-
igation, radio, optical and other electronic equipment,
as well as production of various types of accessories
for such products (electrical boards, magnetic and op-
tical media, etc.). In order to take into account the
influence sub-sectors affiliation on financial perfor-
mance of ICT companies two groups were allocated
in their composition. The first group included enter-
prises dealing with the production of different types of
electronics and components, and the second group in-
volved enterprises producing communication equip-
ment and components.
Based on the form of ownership, most of the com-
panies investigated – 160, companies with limited li-
ability, 16 – is a joint-stock company, 2 – production
cooperative, 1 – limited partnership, 1 – general part-
nership. By type of ownership, the companies investi-
gated are divided as follows: private domestic – 64%;
foreign – 21%; international with a predominant pri-
vate sector – 13%; cooperative – 1%; state – 1%.
Variables. In the research for characteristics of
financial performance of ICT companies were used
four dependent variables – Return on Assets, Net
Profit Margin, Return on Equity, Assets Turnover,
and used in their work by researchers for simiral em-
pirical analysis of the relationship between intangi-
bles values and company financial performance (Gan
and Saleh, 2008; D
ˇ
zenopoljac et al., 2016; Qureshi
and Siddiqui, 2020; Sundaresan et al., 2021; Radoni
´
c
et al., 2021; Serpeninova et al., 2022). For explana-
tion of a relation between intangible assets and finan-
cial performance of ICT companies used intangible
assets variables – Research and Development Inten-
sity, Research and Development Intensity Squared,
Software, Intellectual Property Rights, Acquired In-
tangible Assets. The election of such independent
variable is justified by the financial statements of Slo-
vak ICT companies in the disclosure of information
about intangible assets. As it was revealed Hu
ˇ
nady
et al. (Hu
ˇ
nady et al., 2019), the firm’s ICT sector
account for significant share of total business R&D
expenditure in economy in most countries. There-
fore, in the analysis impact of intangible assets on fi-
nancial performance of ICT sector an important role
should be assigned to R&D indicators. As a result,
the study does not use the indicator of R&D costs
but uses two calculation ratios that characterize the
R&D of the companies. In addition, based on pre-
vious studies (Ievdokymov et al., 2020; Zavalii et al.,
2022; Serpeninova et al., 2022) in our study used three
control variables – Leverage, Size and Dummy vari-
able for ICT sub-sectors. Use of these variables will
allow to control for a significant effects of company
size, level of borrowing capital, and unseen role of
ICT sub-sectors affiliation.
Types, calculation procedures, and abbreviations
used in the Variables study are shown in table 2.
The dynamics of four indicators, that character-
ize financial performance of Slovak ICT companies
(ROA, NPM, ROE, ATO) for the period 2015–2019
showed in figure 3.
Figure 1 displays the change in time of financial
performance indicators for the 2015–2019 period. It
allows to identify a number of common trends: Si-
multaneous growth in all indicators for 2017–2018
years; decrease in ATO, ROA and NPM indicators for
2015–2016 years, their growth in 2016–2018 years,
Empirical Evidence of Intangible Assets Improve the Financial Performance of Slovak ICT Companies
43