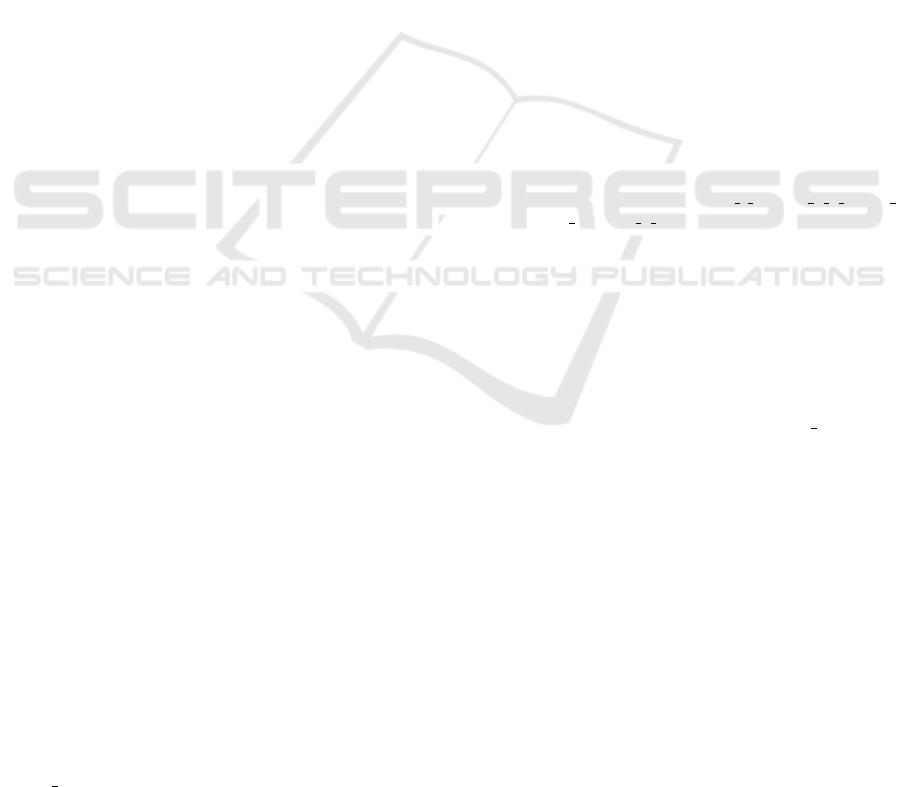
ACKNOWLEDGEMENTS
The work was carried out within the framework of
the program to support scientists from Ukraine dur-
ing the implementation of the project “Mechanism to
strengthen the social responsibility of refugees and
people fleeing the Russian armed conflict on the terri-
tory of Ukraine” on funding from the Polish Academy
of Sciences and the National Academy of Sciences of
the United States and University of Zielona G
´
ora.
REFERENCES
Aquilani, B., Piccarozzi, M., Abbate, T., and Codini, A.
(2020). The Role of Open Innovation and Value Co-
creation in the Challenging Transition from Industry
4.0 to Society 5.0: Toward a Theoretical Framework.
Sustainability, 12(21):8943. https://doi.org/10.3390/
su12218943.
Czy
˙
zewski, B., Poczta-Wajda, A., Kułyk, P., and Drozdz, J.
(2022). Small farm as sustainable nexus of contracts:
understanding the role of human capital and policy
based on evidence from Poland. Environment, De-
velopment and Sustainability. https://doi.org/10.1007/
s10668-022-02485-2.
Czy
˙
zewski, B., Sapa, A., and Kułyk, P. (2021). Human Cap-
ital and Eco-Contractual Governance in Small Farms
in Poland: Simultaneous Confirmatory Factor Anal-
ysis with Ordinal Variables. Agriculture, 11(1):46.
https://doi.org/10.3390/agriculture11010046.
De Felice, F., Travaglioni, M., and Petrillo, A. (2021). Inno-
vation Trajectories for a Society 5.0. Data, 6(11):115.
https://doi.org/10.3390/data6110115.
Dishman, L. (2013). How Free People is Using Big Data
and Social Commerce for Bigger Sales. http://surl.li/
dzmwk.
Dzhedzhula, V., Hurochkina, V., Yepifanova, I., and Tel-
nov, A. (2022). Fuzzy Technologies for Modeling
Social Capital in the Emergent Economy. WSEAS
Transactions on Business and Economics, 19:915–
923. https://doi.org/10.37394/23207.2022.19.80.
Ellitan, L. (2020). Competing in the Era of Indus-
trial Revolution 4.0 and Society 5.0. Jurnal Mak-
sipreneur: Manajemen, Koperasi, dan Entrepreneur-
ship, 10(1):1–12. https://doi.org/10.30588/jmp.v10i1.
657.
Foresti, R., Rossi, S., Magnani, M., Guarino Lo Bianco,
C., and Delmonte, N. (2020). Smart Society and
Artificial Intelligence: Big Data Scheduling and the
Global Standard Method Applied to Smart Mainte-
nance. Engineering, 6(7):835–846. https://doi.org/10.
1016/j.eng.2019.11.014.
Hayashi, N. and Nagahara, M. (2019). Distributed Sparse
Modeling for Society 5.0: Big Data Analysis over
Multiagent Networks. IEICE ESS Fundamentals Re-
view, 13(2):95–107. https://doi.org/10.1587/essfr.13.
2 95.
Hooks, D. L. (1971). Monopoly Price Discrimination
in 1850: Dionysius Lardner. History of Polit-
ical Economy, 3(1):208–223. https://EconPapers.re
pec.org/RePEc:hop:hopeec:v:3:y:1971:i:1:p:208-223.
Hordei, O., Patsai, B., Hurochkina, V., Ovdiienko, O., and
Mishchenko, R.(2021). Optimization of the Invest-
ment Portfolio in the Environment of Table Proces-
sor MS Excel. Studies of Applied Economics, 39(5).
https://doi.org/10.25115/eea.v39i5.4983.
Hurochkina, V., Reshmidilova, S., Bohatchyk, L., Telnov,
A., Skorobogata, L., and Riabinina, N. (2021). Mod-
eling Effectiveness of Financial Support for the So-
cial Capital Development in Economic Emergence.
WSEAS Transactions on Environment and Develop-
ment, 17:262–270. https://doi.org/10.37394/232015.
2021.17.27.
Kitsuregawa, M. (2018). Transformational Role of Big
Data in Society 5.0. In 2018 IEEE International Con-
ference on Big Data (Big Data), pages 3–3. https:
//doi.org/10.1109/BigData.2018.8621989.
Lynch, C. (2008). How do your data grow? Nature,
455(7209):28–29. https://doi.org/10.1038/455028a.
Market Research Future (2022). Healthcare Big Data An-
alytics Market Size, Growth | Industry Trends, 2030.
http://surl.li/dzmwt.
Numa, G. (2016). The monetary economics of Jules
Dupuit. The European Journal of the History of Eco-
nomic Thought, 23(3):453–477. https://doi.org/10.
1080/09672567.2014.951673.
¨
Onday,
¨
O. (2019). Japan’s Society 5.0: Going
Beyond Industry 4.0. Business and Eco-
nomics Journal, 10(2):1000389. https://www.
academia.edu/39149435/Japan s Society 5 0 Going
Beyond Industry 4 0.
Pigou, A. C. (1920). The Economics of Welfare. Macmil-
lan & Co., London. https://oll.libertyfund.org/title/
pigou-the-economics-of-welfare.
Rahmanto, F., Pribadi, U., and Priyanto, A. (2021). Big
Data: What are the Implications for Public Sector Pol-
icy in Society 5.0 Era? IOP Conference Series: Earth
and Environmental Science, 717(1):012009. https:
//doi.org/10.1088/1755-1315/717/1/012009.
SAS Institute Inc (2022). Big Data Analytics: What it is and
why it matters. https://www.sas.com/en us/insights/
analytics/big-data-analytics.html.
Simatupang, A. (2020). Digitalisasi dan Internasionalisasi
Pendidikan Tinggi Dalam Pembentukan Society 5.0
dan Industri 5.0. In Sintha, L., Guswantoro, T., To-
bing, F., Purnamasari, A. A., and Putra, S., editors,
Digitalisasi dan Internasionalisasi Menuju APT Ung-
gul dan UKI Hebat, page 217–218. UKI Press. http:
//surl.li/dzmxm.
Software AG(2022). Event-driven process chain (EPC).
https://www.ariscommunity.com/event-driven-proce
ss-chain.
Tanner, A. (2014). Different Customers, Different Prices,
Thanks To Big Data. https://cutt.ly/q018Ugn.
Valentino-DeVries, J., Singer-Vine, J., and Soltani,
A. (2012). Websites Vary Prices, Deals Based
on Users’ Information. The Wall Street Jour-
nal. https://www.wsj.com/articles/SB10001424
127887323777204578189391813881534.
M3E2 2022 - International Conference on Monitoring, Modeling Management of Emergent Economy
70